Streamlined and scalable CHANGE-Seq method improves understanding of genome editors
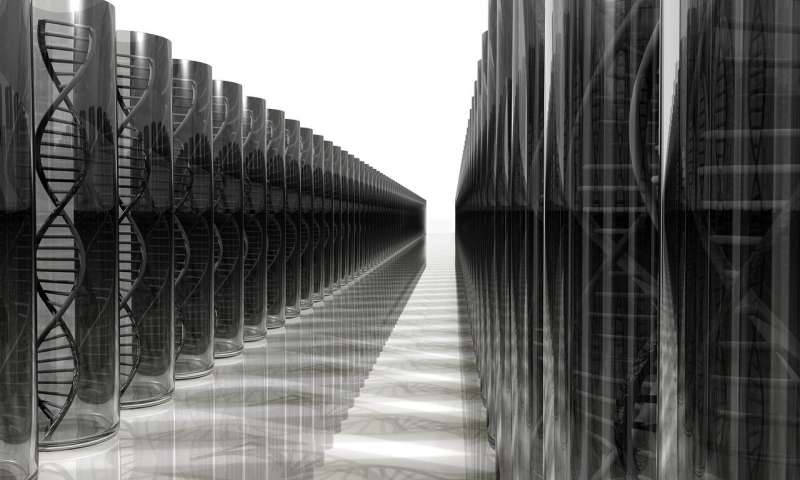
Scientists at St. Jude Children's Research Hospital have developed an easy to use, sensitive and high-throughput method to define sites of unintended double stranded breaks in DNA caused by genome editors like the CRISPR-Cas9 technique. They called the method Circularization for High-throughput Analysis of Nuclease Genome-wide Effects by Sequencing (CHANGE-seq). The work appears as an advance online publication today in Nature Biotechnology.
CRISPR-Cas nucleases are a transformative genome editing technology that has become broadly adopted for research and is being studied as a basis for future therapeutics. While genome editing holds tremendous promise for improving treatment of cancer, sickle cell disease and other conditions, it remains challenging to screen for highly specific targets. Factors that affect unintended off-target activity remain largely unknown.
"CHANGE-seq is the first truly scalable method for illuminating the unintended activity of CRISPR-Cas nucleases," said corresponding author Shengdar Tsai, Ph.D., of St. Jude Hematology. "With this method, scientists can now rapidly pick the best and safest genome editors and targets for therapeutic editing, such as for treatment of sickle cell disease and for cancer immunotherapy."
An individualized tool
Everyone's genome is unique, and these differences can lead people with the same disease to have varied responses to therapy. Therefore, it is important to have methods that can be applied to individual genomes to better study and understand safety.

"CHANGE-seq can be used to help define the effects of human genetic variation on genome editing activity," said first author Cicera Lazzarotto, Ph.D., of St. Jude Hematology. "We found that individual genetic variation can strongly affect unintended genome editing activity in vitro."
The simplicity of CHANGE-seq enables it to be rapidly applied to any source of genomic DNA. Additionally, the capability of CHANGE-seq to analyze many samples enabled the researchers to generate a large dataset that they used to train a machine-learning algorithm to accurately predict unintended activity.
With this resource, they were able to identify simple metrics associated with high-specificity targets for genome editing. The results showed that sites of high activity and specificity are independent, which suggests that using CHANGE-seq makes it possible to find ideal targets with both properties.
"CHANGE-seq enabled us to examine the effects of genetic and epigenomic factors on genome editing activity in human primary T-cells at unprecedented scale," said author Yong Cheng, Ph.D., of St. Jude Hematology.
The CHANGE-seq protocols and open-source analysis software are included in the paper and are available online for other researchers.
Explore further
Comments
Something to say?
Log in or Sign up for free