The company driving wafer scale computing for AI and machine learning applications, Cerebras, has announced a number of system wins in the last 12 months, and counts Argonne National Laboratory, Lawrence Livermore National Laboratory and Pittsburgh Supercomputing Center among its customers. At Livermore, the Cerebras CS-1 machine was recently integrated into the National Nuclear Security Administration’s Lassen supercomputer, the unclassified companion system to Sierra.
Powered by Cerebras’ 1.2 trillion transistor Wafer-Scale Engine (WSE) chip, the CS-1/Lassen union creates what Cerebras CEO and cofounder Andrew Feldman deems a new type of cluster. We spoke with Feldman (virtually) in connection with Hot Chips this week, where the company was discussing software codesign and revealing just a little bit about its next-gen wafer chip technology (more on that below).
“We are the only dedicated AI system fully integrated into a supercomputer, and so our system is now a part of Lassen,” Feldman said. “It allows some of the machine to do simulation, or emulation, and in other parts to integrate AI.”
The CS-1 is part of the workflow, such that AI is baked in. “It doesn’t go to a different place, get done and brought back; it’s in the heart of the environment,” Feldman said.
The work that Livermore is doing on Lassen is predominantly a combination of high performance computing plus AI, where they integrate simulation training and inference for physics material science, optimization of fusion applications and drug development.
Livermore scientists are terming this new style of computing “cognitive simulation” (CogSim).
“While Moore’s Law is not yet dead, we can see that it’s slowing down. The historic cadence of hardware improvements has slowed or even ceased, but the demand for computing has not,” said Chief Technology Officer for Livermore Computing Bronis R. de Supinski, who led the CS-1 procurement effort. “We need new answers for how to improve our ability to meet our mission requirements and to respond to ever-increasing computational requirements. Cognitive simulation is an approach that we believe could drive continued exponential capability improvements, and a system-level heterogeneous approach based on novel architectures such as the Cerebras CS-1 are an important part of achieving those improvements.”
The combined system embodies the trend toward greater heterogeneity, where work is coordinated across specialized computing elements. The result is a more efficient and integrated workflow.
“Having a heterogeneous system is motivating us to identify where in our applications multiple pieces can be executed simultaneously,” said LLNL computer scientist Ian Karlin. “For many of our cognitive simulation workloads, we will run the machine learning parts on the Cerebras hardware, while the HPC simulation piece continues on the GPUs, improving time-to-solution.”
LLNL and Cerebras are partnering on an Artificial Intelligence Center of Excellence (AICoE) to determine the optimal parameters for applying cognitive simulation to the lab’s workloads. Successful outcomes could portend additional CS-1 systems at Livemore, to be used with Lassen or other lab systems.
Lassen was part of the same Department of Energy contract that brought Sierra to Livermore in 2018. Leveraging IBM Power9 CPUs and Nvidia Volta GPUs across 684 EDR-connected nodes, Lassen delivers 15.4 Linpack petaflops and is ranked as the 14th fastest system in the world.
The CS-1 — a 15 RU standard rack-compliant server with 1.2 Tbps I/O provided by 12 x 100 GbE — connects to Lassen via an InfiniBand to Ethernet gateway. The system is programmable via TensorFlow, PyTorch, and other frameworks.
Cerebras offers a competitive comparison with Nvidia’s latest GPU, the Ampere A100. “They went to 7nm, we’re still 54 times more cores and 450 times more on-chip memory, 5,000+ times more memory bandwidth, 20,000 times more fabric bandwidth” Feldman stated.
The company says its 400,000 AI-optimized cores (etched on one wafer-scale die) can do the AI training work of 100-1,000 GPUs. The 15 rack-unit CS-1 has a max power draw of 20 kW; pricing has not been publicly disclosed.
The WSE’s 18 Gigabytes of on-chip memory, 9 petabytes per second memory bandwidth, and 100 petabits/second fabric bandwidth drive its differentiation from other AI hardware products.
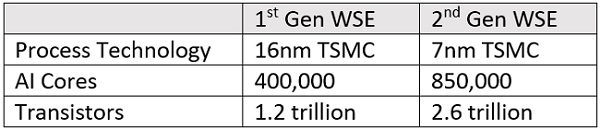
“We are optimized for graph computation,” said Feldman. “And all of neural networking is a subset of graph computation, whether it’s CNNs or RNNs, or LSTMs, or whether it’s the new types of models like BERT or Transformer. That’s our wheelhouse. We’re not optimized for graphics, we’re not optimized for databases, we’re not optimized for web.”
“We’re optimized for this class of work that is heavily dependent on communication among interacting nodes. What’s different between an LSTM or an RNN or CNN is the shape of the communication structure; what they have in common is they’re fundamentally dependent on exceptional inter-core communication, and that’s the hard part of the problem.”
Cerebras was at Hot Chips this week to talk about software co-design for the WSE, a pivot from the company’s original plans to discuss the second-generation WSE architecture. Whether that was due to a shift in timeline or just in the messaging rollout, Cerebras did still provide a sneak peak.
The second-generation Wafer Scale Engine uses TSMC’s 7nm process. Each die has 850,000 AI-optimized cores and 2.6 trillion transistors.
“We have it working in our lab today,” said Cerebras Chief Hardware Architect and Cofounder Sean Lie.
Link to joint announcement from Lawrence Livermore National Laboratory and Cerebras: https://www.hpcwire.com/off-the-wire/llnl-pairs-computer-chip-from-cerebras-with-lassen-supercomputer-to-accelerate-ai-research/
Comments
Something to say?
Log in or Sign up for free